
Responding to Generative AI
The headlines are rife with Artificial Intelligence updates. Some of the articles spell out concerns and fears. Justified concerns if you have ever seen any number of films set in a dystopian future. Others celebrate our inevitable march towards an all knowing Artificial Intelligence (AI). Most miss the nuance of what is general AI, compared to the demonstrated practical applications to date. All the exhibitions remain far from Artificial General Intelligence.

Building A Retention Model
Aristotle declared there to be three primary causes to outcomes. The Efficient (natural), the Formal (momentary, explainable), and the Final (root) cause. Every year countless students start and abandon a formal college education. Each case is unique and should be treated as such when working with an individual student. However, when attempting to design policies and retention tools across the broader student body, we need to find some pattern in the sea of disparate circumstances that cause students to leave and not return. This is one scenario in which data science can help, if used correctly.

General Assembly - Data Science Remote Course
I recently completed a Data Science course via General Assembly (GA). In that course, I learned all of the requisite skills to get started down a path in Data Science, be it for individual curiosity, career pursuits, or both. We were taught the techniques and the tools for everything from data gathering, to cleansing, model selection, feature tuning, and much more. I am grateful for that course and the baseline knowledge that GA gave me in Data Science. I wanted to pursue this course because too many models and algorithms are being created as black boxes with no transparency and lack the needed inclusion of minority voices in their design and feedback. I touch on the need for wide array of voices participating in Data Science in a panel I participated in at the Women Who Code CONNECT Conference.
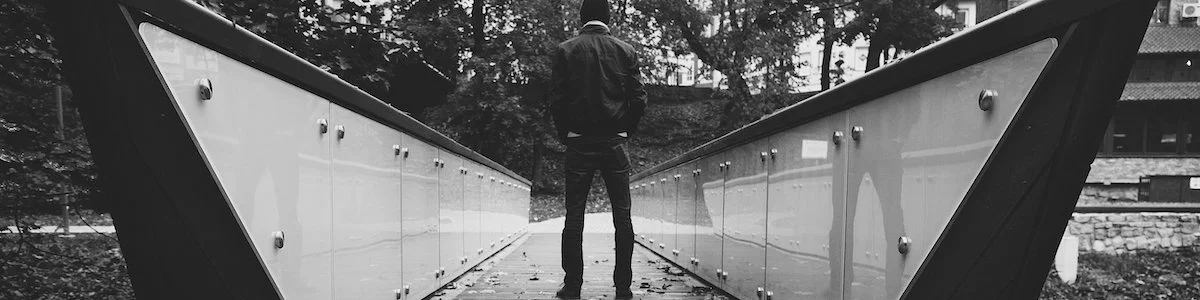
Differential Privacy & Ethics in ML
The dilemma we face in data privacy is determining how does the distribution of control over the models work and what is the correct balance of legislation and counter measures that can be used to control misuse? In a world where greed is the norm, we need to assume that the data of the lower classes will be used against and in favor of them.